Artificial intelligence (AI) is making significant inroads in the field of information technology (IT), thereby altering the prevailing paradigm of IT architecture. While AI possesses substantial analytical capabilities, there remain domains in which enterprise solution architects maintain a competitive advantage, a feat attributable to their unique synthesis of skills, experience, and comprehension of intricate systems. The ensuing discourse aims to offer a concise examination of the impact of AI on enterprise solution architecture (ESA).
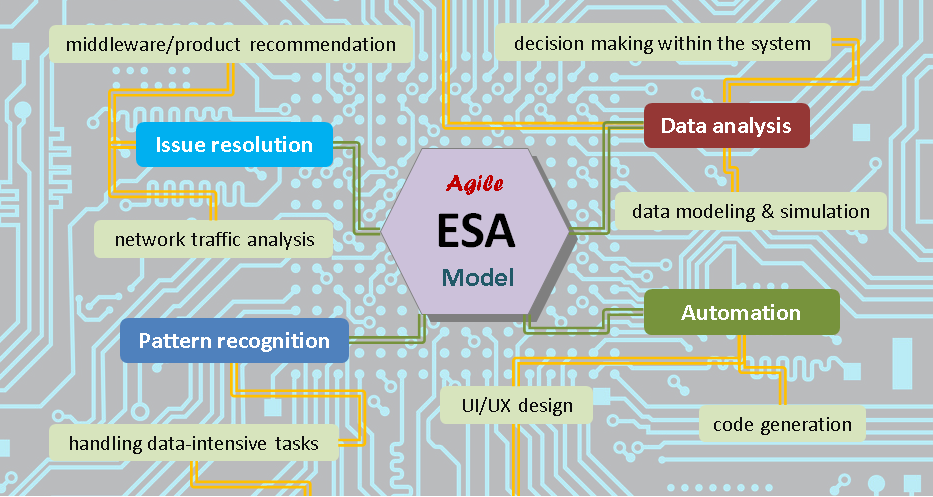
AI-driven intelligence in ESA
AI (or machine learning, or further defined into deep learning) offers unique capabilities to support ESA, including
- Data analysis examples – Prediction, insight and intelligent decision making within the system, data modeling and simulation.
- Issue resolution examples: Using built-in tools and analyzing and recommending myriad middleware, effective network traffic analysis.
- Automation examples: Code generation and UI/UX design
- Pattern recognition examples: handling data-intensive and pattern recognition tasks.
ESA’s role in AI-driven architecture
In AI-driven architecture, ESA architects and human-driven models play a unique role in the following areas:
- Problem Space
- Strategic thinking and long-term planning
- Business alignment and domain expertise, specific business processes and organizational contexts, subtle business logic, etc.
- Case scenario identification
- Requirements and constraints specification for scenario modeling
- Value stream mapping, EA capability and solution service mapping
- Interdisciplinary considerations
- Solution Context
- Architectural thinking about the overall framework and the right level of abstraction
- Novel and unstructured solutions
- Complex enterprise integration methodologies that require a holistic view and experience, such as large manufacturing enterprises
- Constraints and trade-offs in terms of resource allocation and balancing of different applications and systems, including those related to AI.
- Human Intelligence
- Top-down architectural approach that guides initial and customized enterprise solutions
- Regulatory compliance, such as ethical decision-making
- ESA stakeholder involvement, cross-functional team building, holistic views, change management, etc.
- Rare, subtle, and domain-specific solutions, compatibility issues, or unforeseen technical challenges
- Integration of AI algorithms into a data processing system and a unified data management mechanism, such as ensuring that patient data is properly stored and managed.
An effective approach: ESA Model-Driven, AI-Assisted Collaboration
Modern IT architecture uses AI to create a more efficient and effective solution. The most effective approach is likely to be human-AI collaboration, such as AI-driven collaborative filtering (user-based, item-based, or content-based), as seen in some live systems such as Amazon’s recommendation system and Alibaba’s supply chain management system. Key areas of collaboration include
- Goal alignment
- Continuous learning and improvement
- Right level of abstraction
- Keep the model simple enough to understand
- Take an IT service-based approach to modeling
- ESA metrics specification and correlation
- Leverage AI’s knowledge base, analysis and recommendation capabilities
- Determine the fittest solution (often not the best)
- Avoid over-reliance on AI in IT architecture (Note: AI-driven IT architecture decisions can lead to technical debt)
- ESA modeling of architectural integration
- Leverage AI-powered middleware and integration tools
- Choose a hybrid architecture with AI-enabled components
- Address interoperability challenges
- Data-driven decision making
- Collaboratively analyze data to understand system health and issues
- Establish data governance, data policies, and data ownership
When we talk about ESA (Enterprise Solution Architecture), we’re actually referring to agile ESA, also known as A-ESA. This approach focuses on IT service-based modeling. It’s important to remember that an AI-assisted ESA will only be as good as the agile practices it’s paired with.
ESA architect’s key skills in AI-assisted solutions
Simply put, ESA architects need to stay on top of AI trends and understand AI capabilities. Be sure to:
- Set clear goals and AI-enabled metrics, and design flexible architectures
- Ensure data compatibility
- Foster collaboration with AI experts
- Integrate AI components and capabilities into ESA modeling to facilitate key IT architecture considerations that matter for sustainability
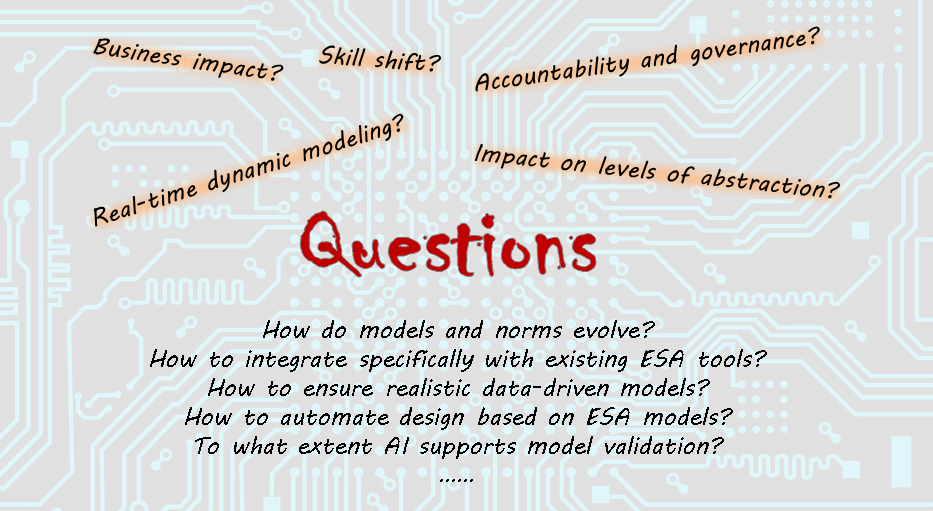
Conclusion
The future trends in the use of AI in IT architecture are obvious. There will be deeper integration with cloud computing, full stack automation from development to operations, AI-driven security defense, continuous optimization of system performance, improved system reliability and resilience, etc. More importantly, there is a growing need to place a greater emphasis on enhancing the explainability of AI decisions within the context of IT architecture, intelligently integrating architecture across heterogeneous systems, and improving AI-architect collaboration.
While EA (unless it’s really meant by ESA) is mostly a strategic planning tool, system or solution design is more focused on technology or implementation. Neither fits the holistic thinking achievable in the ESA space.
EA will become much easier as AI capabilities grow, and so will solution design. However, ESA, the tough part that connects both IT strategies and solution landing, is still primarily up to architects rather than AI bots, because architectural trade-offs are mostly human decisions, especially at the enterprise application level. In addition, it’s the ESA architects who determine the right level of abstraction and integration styles.
Looking ahead, there will be more collaboration between AI and IT architects in large solutions through an IT service-based architectural approach. EA will serve as a strategic direction like IT principles, and composite design will serve as services. Powered by zooming intelligence, the Agile ESA model will become both a blueprint and a steering dashboard for the AI-driven solution/platform model that is most helpful to IT architectural thinking. Until then, EA will truly connect the real world of IT, and ESA will become a better composable or utility service-based architecture.
Checking…
Retry »
Sending message…
Let’s Connect
Fill in the form below and we will be in touch soon